Today, Artificial Intelligence (AI) has swept into every aspect of the our lives, with the AI applications like ChatGPT and Sanpchat’s My AI Snaps attracting significant attention. Generative Pre-trained Transformer (GPT) is a deep learning model that uses a large-scale neural network to generate natural language texts. GPT is based on the transformer architecture, which consists of multiple layers of self-attention and feed-forward modules. GPT can learn from a large corpus of unlabeled text data and then fine-tune on specific tasks such as text summarization, machine translation, question answering, and more.
One of the main goals of GPT is to achieve artificial general intelligence (AGI), which is the ability of a machine to perform any intellectual task that a human can do. To this end, GPT aims to capture the common sense knowledge and reasoning skills that are implicit in natural language texts. GPT also strives to generate coherent and diverse texts that can satisfy various user intents and preferences.
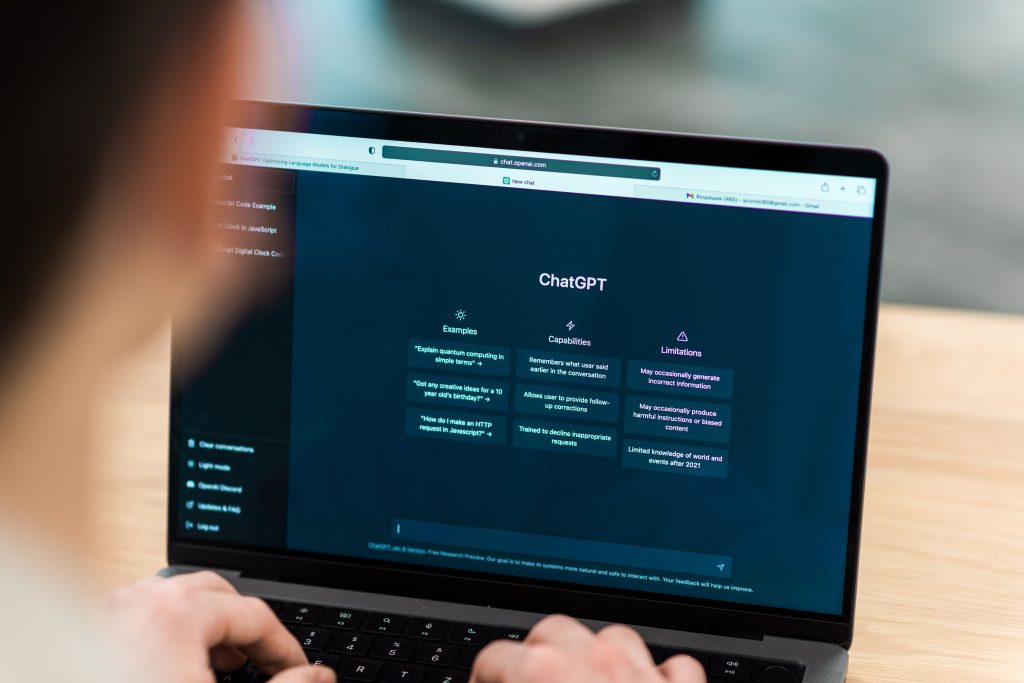
Some of the key features and challenges of GPT are:
• AGI-GC: AGI-GC stands for artificial general intelligence via generative capsules, which is a framework proposed by OpenAI to achieve AGI using generative models. AGI-GC suggests that generative models can learn to represent and manipulate complex concepts by composing simpler ones in a hierarchical manner. Generative capsules are the building blocks of this framework, which are neural modules that can generate and encode information in a latent space.
• Model Parameter: Model Parameter refers to the size and complexity of GPT models, which can have billions of trainable parameters in their neural networks. For example, GPT-3, the latest version of GPT, has 175 billion parameters, making it one of the largest models ever created. Billion level parameters pose challenges for training, inference, and deployment of GPT models, such as computational cost, memory consumption, scalability, and robustness.
• Large Language Model(LLM): A large model is a type of artificial intelligence algorithm that can process and understand human languages or text using neural network techniques with lots of parameters1. A large model is usually trained on vast amounts of text data, mostly scraped from the Internet2. A large model can perform various tasks such as recognizing, summarizing, translating, predicting and generating text and other forms of content.
• Reinforcement Learning: Reinforcement learning (RL) is a machine learning paradigm that involves learning from trial and error, based on rewards and penalties. RL can be used to train GPT to generate texts that optimize certain objectives or metrics, such as fluency, relevance, diversity, or user satisfaction. RL can also be used to explore different strategies or policies for text generation, such as sampling methods, decoding algorithms, or temperature parameters.
• Emergence: Emergence is the phenomenon where complex patterns or behaviors arise from simple interactions or rules. Emergence can be observed in natural language texts, where meanings and structures emerge from combinations of words and sentences. Emergence can also be observed in GPT-generated texts, where novel or creative texts emerge from combinations of tokens and layers. Emergence can be beneficial for GPT models, as it can enhance their expressiveness and diversity.
• RLHF: RLHF stands for reinforcement learning with human feedback, which is a method to improve the quality and diversity of GPT-generated texts by incorporating human judgments. RLHF involves collecting human feedback on the generated texts, such as ratings, preferences, or corrections, and then using them as rewards or penalties for training the model. RLHF can help GPT learn from human preferences and expectations, as well as avoid generating harmful or inappropriate texts.
• Generalization: Generalization is the ability of a model to perform well on unseen or new data or tasks, after being trained on a limited or specific set of data or tasks. Generalization is an important goal for GPT models, as it indicates their adaptability and robustness. Generalization can be achieved by using large and diverse datasets for pre-training, as well as using effective methods for fine-tuning or transfer learning.
• Fine Tuning: Fine tuning is a method to adapt a pre-trained model to a specific task or domain, by updating its parameters with a small amount of labeled data. on downstream tasks or domains that require specialized.
GPT can Assist to Improve Your Business Efficiency
• Automating routine tasks such as content creation, data analysis, document generation, and more.
• Understanding and communicating with customers effectively by generating natural and engaging responses, providing personalized recommendations, and offering multilingual support.
• Streamlining internal processes such as project management, knowledge sharing, training, and collaboration by generating summaries, reports, feedback, and suggestions.
• Gaining valuable insights from large and complex data sources by generating visualizations, interpretations, predictions, and scenarios15.
Kingdee Released the CosmicGPT
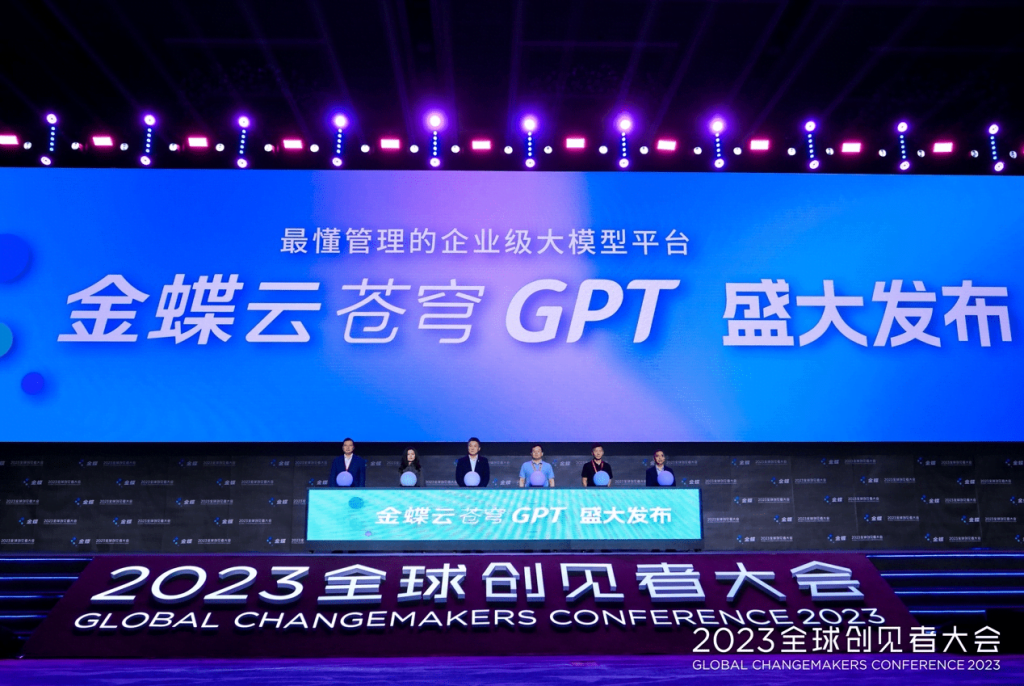
On August 8, Kingdee held the “2023 Global Creators Conference” in Shenzhen on the occasion of its 30th birthday. During the conference, Kingdee released the CosmicGPT large model with tens of billions of parameters. CosmicGPT large model is based on the continuous pre-training of general large models and is positioned as an enterprise-level large model platform that understands management.